End unplanned downtime: Best practices for asset failure mitigation
From the power generation and chemicals industries to manufacturing and paper, use cases paint dramatic portraits of unplanned outages averted with the help of predictive analytics software. US-based utility Duke Energy saved over $34 million in a single early warning catch. SCG Chemicals increased plant reliability from 98% to 100% by providing early identification and warning of potential asset failures before they occur. Headline-grabbing stories like these are becoming increasingly common as more and more industrial enterprises embrace the benefits of early warning detection of anomalies and fault diagnostics.
Recent forecasts suggest the global market size for predictive analytics will grow from roughly $12.5 billion in 2022 to $38 billion by 2028, representing a 20% compound annual growth rate.1 Such favorable outlooks take into account improved artificial intelligence (AI) and machine learning (ML) technologies and algorithms, fresh approaches to deploying predictive analytics at scale, and the usability and maintainability of the data and systems.
Enterprises weighing a potential deployment of predictive analytics tools must take into account a number of variables, like return on investment (ROI), whether the digital tools they ultimately choose are hardware agnostic and best suited to leverage existing software investment, the level of contextualization the digital solution provides, and how easy the solution is to deploy, maintain, and scale.
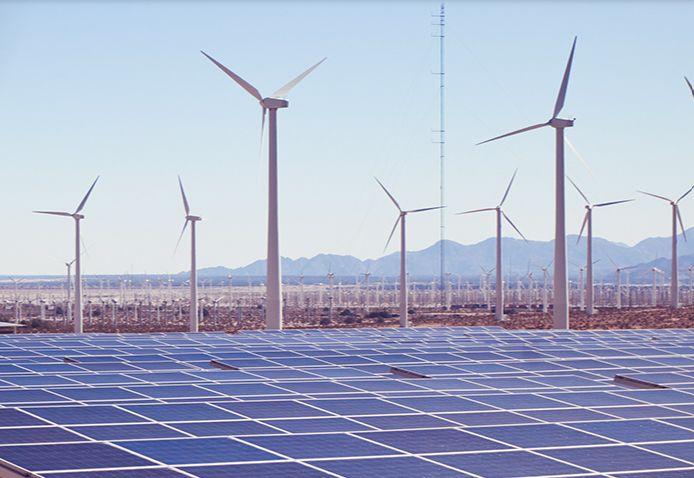
Harnessing the power of human insight
According to a recent study, 70% of operators are unaware of when to replace, upgrade, or maintain assets. While many predictive analytics solutions can deliver anomaly alerts, without the right context, users can easily underestimate the urgency of problems–with potentially detrimental outcomes. A user’s decisionmaking ability is only as good as the insights at their disposal. Even if operators have data at their fingertips, turning that data into meaningful insights also relies on personal experience.
Human experience and intuition have an important role to play in assessing potential asset faults and failures, but depth of experience can vary widely based on a user’s specific area of expertise–whether they come from an engineering or operations background, for example.
Companies must harness the power of human insight while acknowledging that a user’s ability to interpret the criticality of fault data is often subjective. To capitalize fully on their teams’ expertise industrial companies should seek predictive analytics solutions that provide much-needed context around AI-driven predictions.
Any closed-loop predictive analytics strategy must include digital tools that both leverage real-time data to provide timely fault diagnostics alerts and provide users with the appropriate context to interpret those alerts. A closed-loop predictive analytics strategy gives enterprises the ability to collect, organize, and analyze data, including real-time sensor, historical operations, and financial impact analysis data. They can then create meaningful insights with fault diagnostics, prescribe mitigating actions, and use and log best practices for continuous improvement.
The importance of fault diagnostics
Alert management, however, is not without its challenges. On average, sensor failures account for 25% of alerts, degrading the accuracy of analytics. Unreliable data can cloud analysis and decision making. Organizations need clarity and precision to accurately determine if a situation matches asset fault conditions and how individual sensors are contributing to these faults.
Predictive diagnostics uses customized data and diagnostic tools to provide precise, real-time insights and identify potential issues. Best-in-class fault diagnostics account for the estimated time until a failure is likely to occur, helping to accurately prioritize the repair. This time-to-failure forecast helps operations and maintenance teams determine whether to operate an asset until the next planned maintenance outage or initiate an urgent shutdown. This also enables teams to anticipate potential supply chain issues much more accurately and consider lead time for spare parts.
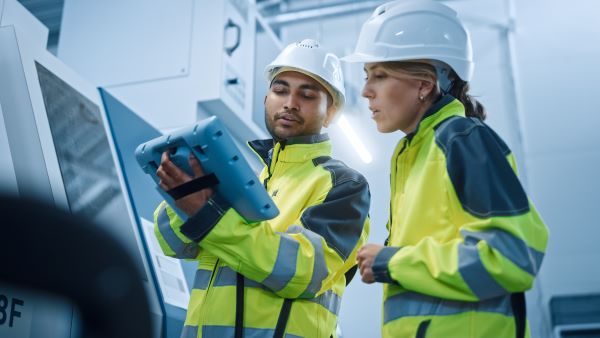
Prescriptive analytics deliver actionable tasks to remediate the problem. This way, unplanned downtime is eliminated. Conversely, it can help an operator determine whether a planned maintenance task can be postponed. Plant personnel can schedule maintenance and assess risk more effectively, helping an organization prioritize safety and profitability.
Getting the most out of data
Industrial companies are collecting more data than ever before. An estimated 50% of all industrial data was created in the last two years alone. According to Seagate, approximately 43% of all collected industrial data remains largely unused. To maximize their data usage, enterprises must make it easy to not only share and access information but, more importantly, to contextualize it with meaningful insights. Many industrial companies struggle to share timely, accurate insights across their global ecosystem. They also find it difficult to efficiently leverage the right data for their predictive analyses.
An organization might already monitor when its assets reach a certain threshold, such as temperature, heat rate, fuel consumption, power consumption, or differential pressures.
While these metrics may represent valuable insights, they are static and, as such, are most useful when operating conditions and load do not change. When conditions do change, operators need multiple parameters to track any deviations in expected asset behavior.
A more dynamic production environment, in which operating conditions like load might vary, demands a more dynamic procedure.
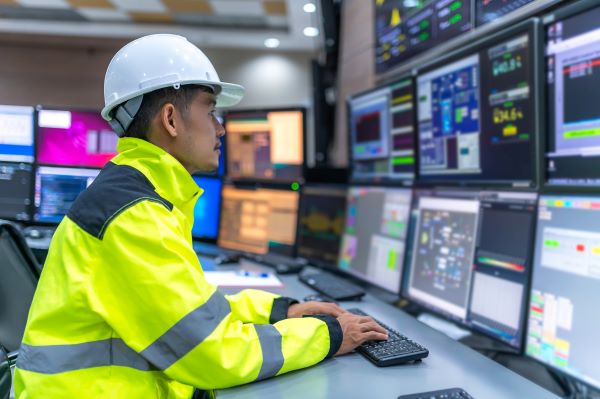
Predictive analytics tools track the actual conditions of assets alongside real-time operating conditions to predict a possible problem in the future. Predictive analytics tools account for multiple thresholds and patterns of change by analyzing historical behavior.
This enables much more effective maintenance plans, eliminating over-maintenance of assets, and delivers a clear view of the relationships between asset models, fault conditions, failure modes, sensors, and actual fault match information to create meaningful insights. This enables users to see fault relationships in great detail to better understand why an asset is degrading and make better risk assessments on maintenance intervention.
To leverage data for maximum impact, companies must build a data infrastructure using solutions that can integrate all sources of pertinent information, from engineering to operations to asset management to enterprise finance. Truly impactful fault diagnostics solutions should be able to both signal an alert and translate that alert into time-to-failure, criticality, and urgency, as well as the economic impact of a failure, while taking into account all necessary parameters from engineering, asset management, and finance to create those insights.
The AVEVA difference
AVEVA’s portfolio of predictive analytics solutions has quickly become the industry standard.
By combining digital twin technology with AVEVA™ Predictive Analytics, companies from a wide range of industrial sectors have forged a path toward improved operations.
When enterprises build a digital replica of an asset or plant using digital twin solutions, they can quickly analyze multiple data sources, eliminate data silos, reduce data complexity, and incentivize collaboration and innovation. When deployed with AVEVA Predictive Analytics and AVEVA™ PI System™ to gain early warning notification and diagnosis of equipment issues, companies regularly see a reduction in operating expenses (OPEX) of up to 20%.
AVEVA Predictive Analytics is a no-code solution that does not require the support of software engineers or data scientists. The AI in AVEVA Predictive Analytics offers advanced alert and case management, enabling knowledge capture and reporting. Templates accelerate configuration, deployment, and scale-up, ensuring maximum ROI. Best-in-class fault diagnostics can identify the most probable failure mode.
Forecasting gives users insight into the remaining time before an asset reaches critical failure and its urgency level. An asset library, derived from over 22,000 manhours of experience, prescribes remediating actions, thereby decreasing repair time.
For example, Mitsubishi Power used predictive analytics solutions from AVEVA to increase the operational awareness of its energy systems. With its newfound capacity to monitor the latest power generation systems, such as hydrogen producers and energy storage batteries, Mitsubishi Power made impressive gains toward its goal of preventing unplanned shutdowns.